O. Sorba and C. Geissler. Advestis Working Paper, May 2021.
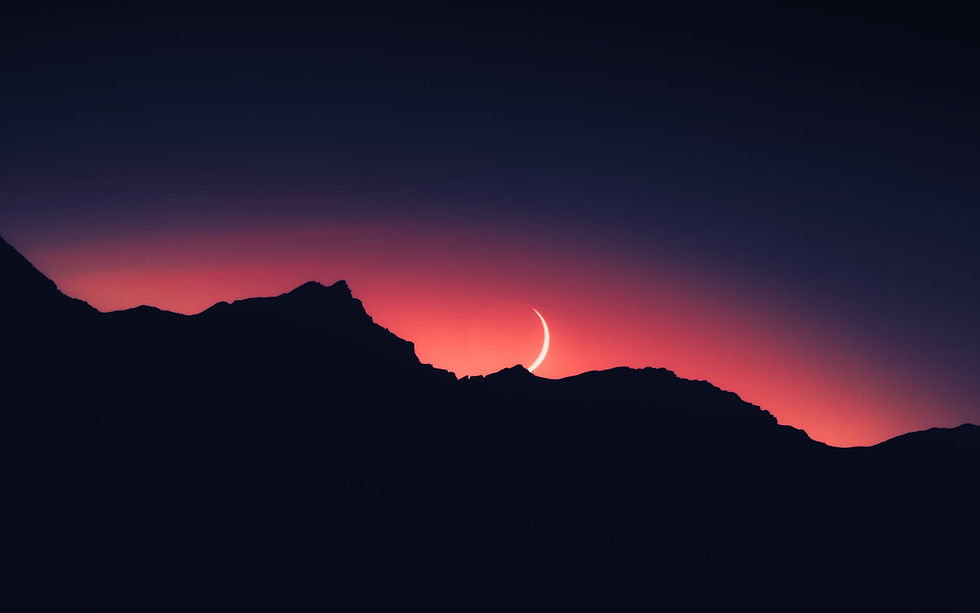
Abstract: The aim of the present study is to detect abrupt trend changes in the mean of a multidimensional sequential signal. Directly inspired by papers of Fernhead and Liu ([4] and [5]), this
work describes the signal in a hierarchical manner : the change dates of a time segmentation process trigger the renewal of a piece-wise constant emission law. Bayesian posterior information on the change dates and emission parameters is obtained. These estimations can be revised online, i.e. as new data arrive. This paper proposes explicit formulations corresponding to various emission laws, as well as a generalization to the case where only partially observed data are available. Practical applications include the returns of partially observed multi-asset investment strategies, when only scant prior knowledge of the movers of the returns is at hand, limited to some statistical assumptions. This situation is different from the study of trend changes in the returns of individual assets, where fundamental exogenous information (news, earnings announcements, controversies, etc.) can be used.
Comments