V. Margot, Towards Data Science, February 17th, 2021.
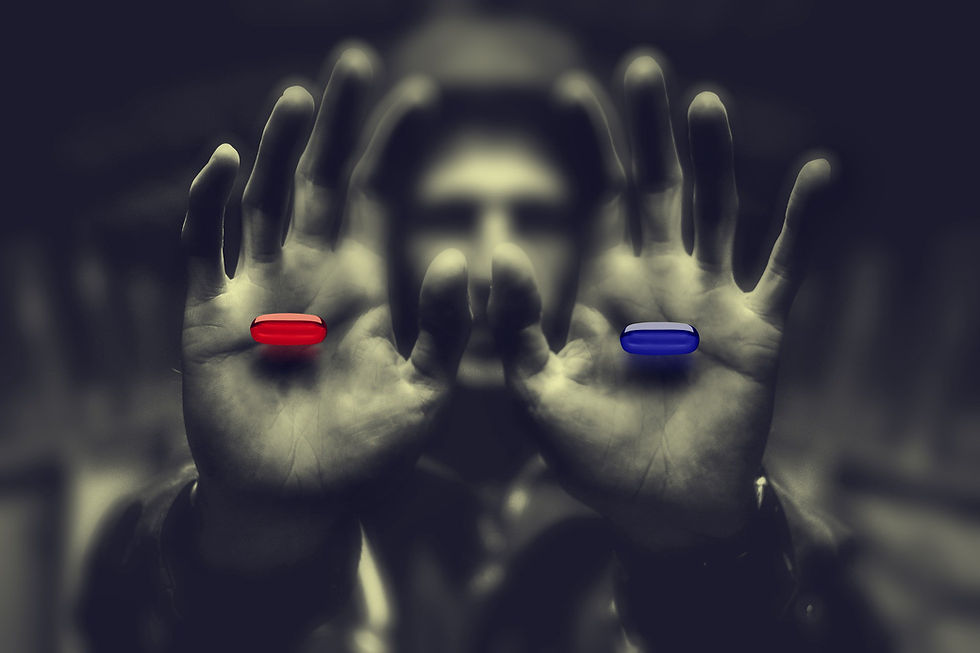
Abstract: When one wants to build an interpretable model, one usually hesitates between the two main families of intrinsically interpretable algorithms: Tree-based algorithms and rule-based algorithms. One of the main differences between them is that tree-based algorithms generate trees with disjoint rules, which has shortcomings such as the replicated subtree problem, while rule-based algorithms generate sets of rules and allow overlapping rules which may be a problem. Indeed, how can you make a decision when two (or more) rules are activated simultaneously? This is even more of a problem when the predictions of the rules are contradictory.
In this short article, I present two methods for obtaining a single prediction from a set of rules, less trivial than an average of predictions, but preserving the interpretability of the prediction.
Comments